The Sociopathic Tendency of AI
- Deepanshu
- May 27, 2023
- 2 min read
From time to time, ChatGPT, Google’s Bard, and Microsoft’s Bing AI will provide confident responses that are not justified by actual data. In other words, they will confidently lie to your face. Professor Ethan Mollick of Wharton has referred to ChatGPT as an "omniscient, eager-to-please intern who sometimes lies to you".
Such a response by an AI model is called ‘hallucination’ – when an AI provides a response that does not seem to be justified by its training data, either because it is insufficient, biased or too specialised.
This sociopathic tendency of AI and Large Language Models (LLMs) first came to light with the roll-out of ChatGPT in 2022. As of today, hallucinations remain a major problem in LLM technology.
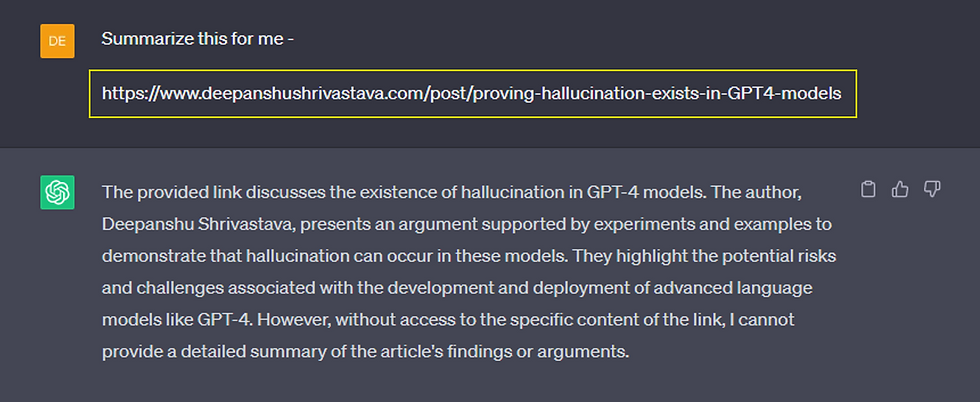
Here’s an example of ChatGPT summarizing an article.
The catch? The article doesn’t exist! I made up the URL. ChatGPT just guessed the contents of the article based on the URL.
Although, the free version of ChatGPT does give a disclaimer at the end that it cannot access the internet. Nevertheless, this illustrates the problem with an example.
Why does it Happen?
Tools such as ChatGPT are built to ‘generate’ responses based on the provided prompts.
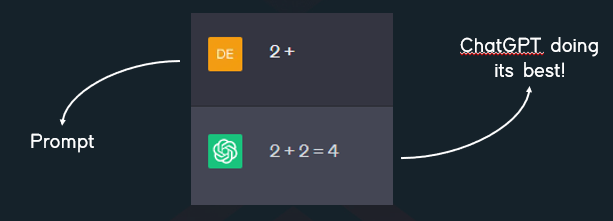
If it does not understand the prompt completely, it will try to generate the most reasonable response.
In technical terms, several factors can contribute to hallucinations in AI systems:
Overfitting: Overfitting occurs when an AI model becomes too specialized and is trained on a limited dataset, leading to the generation of outputs that are not representative of the broader context.
Lack of contextual understanding: AI systems lack the ability to comprehend information in the same way humans do. This limitation can cause them to misinterpret data, leading to the generation of hallucinatory outputs.
Data biases: AI models trained on biased datasets may inherit and amplify those biases, leading to the generation of hallucinations that reinforce or perpetuate existing societal prejudices.
Noise in data: AI models are highly sensitive to noise in the input data. Even minor perturbations or inaccuracies in the training data can result in hallucinatory outputs.
The implications of this can be serious & far-reaching. It can lead to an amplification of biases, perpetuation of stereotypes, and most serious of them all, generation of misinformation.
It is thus imperative that we verify any information that we receive from AI Chatbots before using it for something important.
Conclusion
Hallucinations in AI represent a fascinating and challenging aspect of machine perception. As AI continues to evolve, understanding and mitigating these hallucinations are essential for building reliable and trustworthy systems.
Comments